摘要:人们喜欢用分子对接预测化合物的结合亲和力以解释化合物的活性差异。经常有人吐槽:对接对化合物活性排序非常不准。因此本文的主要目的是探讨分子对接能否精确地预测结合亲和力。本文收集了分子对接软件GOLD与GLIDE的在线支持文档、科技文献以及最近的D3R竞赛结果来说明这个问题。
肖高铿/2019-05-01
前言
分子对接常用于:(1)预测结合模式(pose/binding mode);(2)预测结合亲和力(binding affinity);(3)虚拟筛选(Virtual screening)。经常听到这样的吐槽:我用分子对接预测了化合物的活性,发现对化合物活性排序非常不准。这与分子对接预测结合亲和力相关,因此本文的主要目的是探讨分子对接能否精确地预测结合亲和力。分子对接预测结合模式预测与虚拟筛选的话题另有讨论,见本文的相关主题部分。
用分子对接软件GOLD预测结合亲和力
GOLD是一款著名的分子对接软件,CCDC的在线支持解答了如何用GOLD预测结合亲和力这个问题,原文如下(2019-04-30访问):
GOLD has been optimised for the prediction of ligand binding positions rather than the prediction of binding affinities. It is not recommended to use scoring function values to suggest accurate binding affinities because many factors are ignored or approximated when calculating a docking score (protein and ligand motion, and accurate treatment of waters to name two).
Sometimes a correlation between fitness score and binding affinity can be observed. If so, care must be taken to ensure this is not artifactual. This usually occurs when an equally good correlation is found between binding affinity and molecular weight. Both fitness score and binding affinity tend to correlate with molecular weight to some extent.
It is possible to develop Quantitative Structure Activity Relationships (QSAR) between docking data and binding affinity using GoldMine. These can be used to predict binding affinity for new members in the same series.
For further information please refer to the GOLD and GoldMine user manuals.
大意是:设计GOLD的目的是预测结合模式而不是结合亲和力。不推荐用打分值精确地预测化合物的结合亲和力,因为打分的时候忽略了多个因素或者说进行了粗略地计算(比如忽略了蛋白与配体的运动,水分子的处理等)。有时观察到打分值与结合亲和力之间具有相关性,如果这样的话,一定要非常小心以确保这不是人为的结果。具有相当好的相关性出现时通常也会伴随着结合亲和力与分子量也具有相当好的相关性。在某种程度上,打分值与结合亲和力都趋向于与分子量呈相关性。用GoldMine可以在分子对接数据与结合亲和力之间建立QSAR模型来预测同系物新分子的结合亲和力。
用分子对接软件GLIDE预测结合亲和力
Schrodinger的knowledge base有两个关于如何用Glide预测结合亲和力的解答。其中一个是如何用GlideScoer预测结合亲和力:
Article ID: 572 – Last Modified: May 19, 2016 – 12:00am
How do I find the binding affinity from the GlideScore?
The GlideScore is an estimate of the binding energy, but it is only an estimate. Computing accurate absolute, or even relative, binding energies is an extremely challenging task. While the GlideScore has been fit to experimental binding energies (but for native redocking only), and therefore is on the scale of binding energies (in kcal/mol), it isn’t possible to use the GlideScore for rank-ordering actives. The GlideScore generally can distinguish actives from inactives (e.g., nanomolar vs micromolar) in a virtual screening application, but the use of a rigid receptor structure means that it is not possible to distinguish fine levels of activity, because the particular receptor conformation used for docking might not be optimal for binding all actives. GlideScore differences of a few kcal/mol really can’t be considered significant, which makes it problematic to compare actives.
大意是:GlideScore是对结合能的估计,但它仅仅是个估计。计算精确的绝对或相对结合能是一项极具挑战性的任务。虽然GlideScore已经用实验结合能拟合过,但仅限于redocking(一钟结合模式预测任务)的场合。因此在结合能的尺度上(以kcal/mol为单位),不可能用GlideScore对活性进行(正确地)排序。GlideScore通常用于虚拟筛选中以区分活性和非活性化合物(例如,活性nM对非活性μM的区分度)。分子对接计算采用刚性受体结构意味着不可能对活性区分地很精细,因为用于对接的特定受体构象不可能对所有活性化合物来说都是最佳的。GlideScore具有几个kcal/mol的差异确实不能被认为是显著的,这使得用对接打分值来比较活性是有问题的。
另一个问题是:为什么对接打分与GlideScore与活性没有相关性,哪里出错了呢?原文如下:
Article ID: 144- Last Modified: May 19, 2016 – 12:00am
GlideScore/Docking Score doesn’t correlate with my known activities. What is wrong?Glide is primarily concerned with generating an accurate pose for each protein-ligand complex and separating ligands with appreciable binding affinity (generally < 10uM) from those that don't bind, in a ranked list. Extensive testing, both in-house and by third parties, has shown that Glide is very effective at pose prediction and enriching hit lists in active compounds.
The task of accurately estimating protein-ligand binding affinities remains beyond the capabilities of docking scoring functions. The rigid receptor approximation, limited estimate of the entropy gain or loss upon binding, and other approximations in GlideScore and all other empirical scoring functions omit essential thermodynamics of the free energy of binding. Thus, approaches other than correlation with GlideScore should be applied to predict relative or absolute binding affinities.
- For congeneric series, where a set of ligands share a common structure, free energy perturbation with FEP+ has been demonstrated to reliably produce binding affinity predictions with approximately 1 kcal/mol accuracy.
- End-point approaches have demonstrated in some cases the ability to yield reasonable correlations with experimental binding affinities. Prime MM-GBSA was designed to process output from Glide and returns relative free energy estimates of binding using a Generalized Born solvation model.
- QSAR-based approaches using 3D information from aligned ligand poses in the binding pocket (such as Field-Based QSAR) are sometimes able to identify and explain correlations with experimental binding affinities.
- Finally, with a set of actives and compounds that don’t bind it may be possible to create your own scoring function by fitting a model to experimental binding affinities using AutoQSAR.
大意是:Glide主要涉及为每个蛋白质-配体复合物生成精确的结合模式(pose),并将具有显著结合亲和力(通常小于10uM)的配体与不结合的配体分离。来自内部和第三方的测试表明,Glide对结合模式预测和富集活性化合物方面非常高效。
精确预测蛋白-配体结合亲和力的任务依然超出了对接打分的功能范围。刚性受体的近似、对结合过程的熵增或损失的有限估计、以及GlideScore和其他所有经验打分函数的其他近似忽略了结合自由能的必要热力学成分。因此,不应该用GlideScore,而应该用其它方法来预测相对或绝对结合亲和力。其它方法包括:
- 对于一组具有相同结构的同系物,已证明用FEP+的自由能微扰可以可靠地预测结合亲和力,预测精度约为1kcal/mol。
- 在某些情况下End-point法预测的结合亲和力与实验的结合亲和力具有合理的相关性的。Prime MM-GBSA可以在Glide的输出基础上使用广义Born求解模型预测相对结合自由能。
- 用在结合口袋里的结合模式对配体进行叠合,用该3D信息进行QSAR分析(例如基于场的QSAR)有时能够识别并解释与实验结合亲和力的相关性。
- 最后,使用一组活性化合物物质和一组不结合的非活性化合物,用AutoQSAR将实验结合亲和力拟合于一个模型中,创建自己的打分函数。
总的来说,Schrodinger关于用分子对接预测结合化合物亲和力并排序的答复与GOLD基本一致:对接打分函数设计用于预测结合模式、而不是精确预测结合亲和力。如果要精确预测结合亲和力,需要另找其它方法,比如用对接产生的pose进行FEP计算,最为精确; MM-GBSA有时有相关性;用对接的pose进行叠合建立QSAR模型也行;还可以用AutoQSAR拟合自己的打分函数。
Enyedy与Egan等人(2008)的研究1
Enyedy & Egan等人(2008)1研究了分子对接打分值与IC50的关系,结果表明GLIDE对接打分值与IC50没有关系,见图1。这与GOLD,GLIDE的描述基本一致。
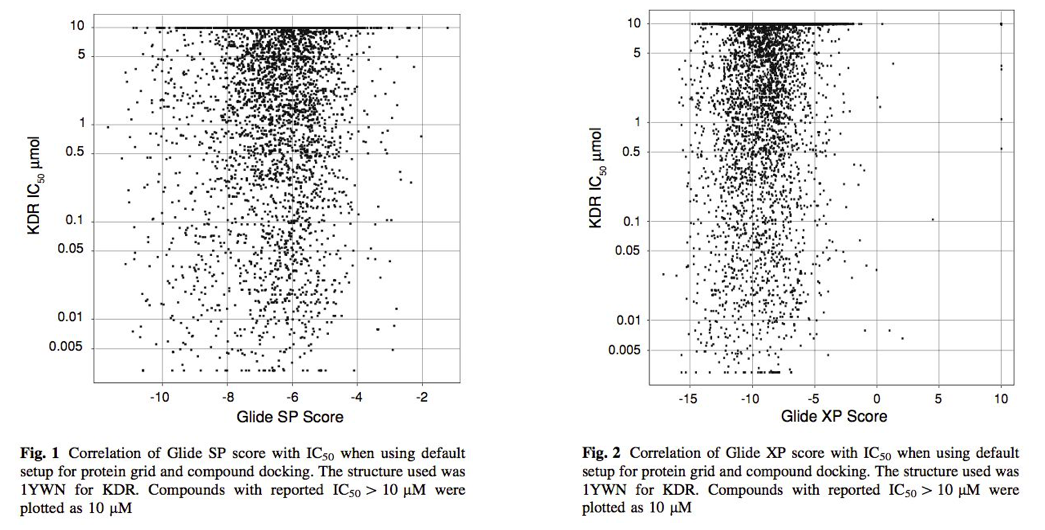
Figure 1. GLIDE SP与GLIDE XP打分值与IC50的关系
此外,还发现有时对接打分通常与分子量、油水分配系数有很强的相关性,甚至MW、ClogP与分子对接打分值一样具有相似的虚拟筛选性能,见图2。这个结果与GOLD关于打分值与分子量关系的描述是一致的。
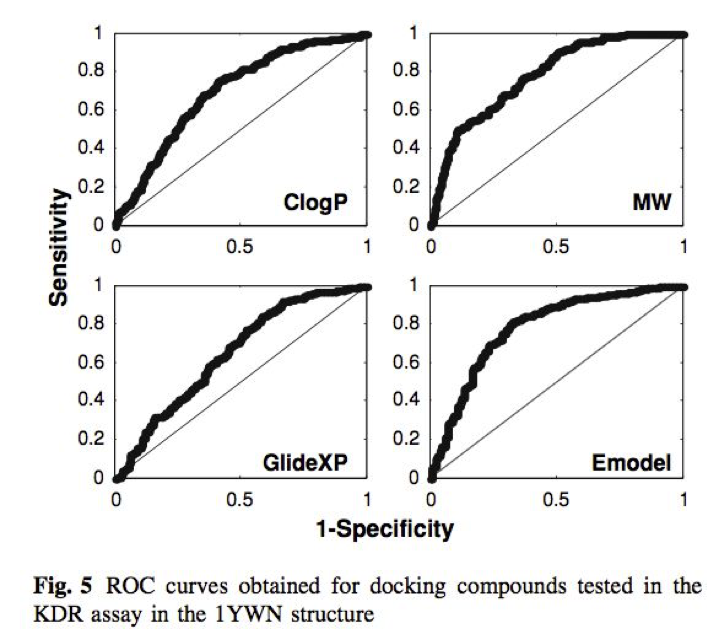
Figure 2. GLIDE_XP与Emodel打分函数与ClogP与MW的虚拟筛选性能比较
总的来说,分子对接打分用于hit-2-lead的时候,需要专家对对接结果进行解读,而不能只看打分值。
UCSF DODC6使用手册对Docking score的说明
UCSF DOCK6的常见问答(FAQ)里也回答了打分函数与分子结合亲和力这个问题:
What is a DOCK Score ?
…
http://mailman.docking.org/pipermail/dock-fans/2005-February/000035.html…
DOCK and other docking algorithms are designed to rank libraries of molecules with respect to each other. If this is the level of accuracy you need, DOCK should work fine. If you would like to calculate the absolute binding free energy, you will need to move to much more sophisticated (and time intensive) calculations. Some of the more commonly used of these types of calculations include MM/PBSA, Free Energy Perturbation (FEP), and Thermodynamic Integration (TI). You should be able to find some literature reviews that cover the strengths and weaknesses of each technique.
DOCK的作者之一John Irwin对预测值与实验值有相关性这件事的回复原文:
Thanks for your contribution to the DOCK developers’ discussion group. We welcome all comments and opinions! Arguably this thread fits more in with the dock-fans mailing list, so I’ve copied them on this.
I wonder whether there is a misunderstanding about molecular docking and virtual screening lurking behind what you’ve written. In our experience, molecular docking (virtual screening in high throughput) is considered to be doing well retrospectively if it can a) enrich known binders 20 fold over random from a database of drug-like decoys and b) reproduce qualitatively the experimental binding geometries (McGovern & Shoichet, J Med Chem. 2003 Jul 3;46(14):2895-907.)
Prospectively, we consider docking a success if we purchase and test 50 compounds from among the top 500 of a database of purchasable, drug-like compounds (e.g. ZINC http://zinc.docking.org/ ) and find 3 previously unknown binders. That’s a pretty low bar, but it is considered the state of the art in this field. If someone shows me a quantitative comparison between docking energies and experimental binding affinities, unless it is within a narrow SAR series (and therefore not very interesting), my instinct is to believe it is an accidental correlation, and that people are fooling themselves intobelieving the correlation is significant.
You can list a dozen reasons why docking shouldn’t even work, much less provide good correlations with experimental binding affinities. Indeed, in our experience, 90+% of top docking hits are not actual binders. Correlate that! Hardly worth repeating to this audience, the reasons docking shouldn’t work include but are not limited to the approximations of the scoring function, the inadequate treatment of desolvation and entropy, and the rigid or incomplete sampling of receptor structure.
We think of docking as a screen, that sorts a database into “more likely” (top scorers) and “less likely” (the rest) to actually bind experimentally. Of course, we are actively working to improve docking, and there is reason to hope that docking can be improved. One way to do this is to focus on the decoys, and ask what makes molecules score well in the computer when they do not bind experimentally. This is one area of research in the lab, and the subject of a paper that will appear shortly from Graves and Shoichet 2005.
You are right to be cautious, and I encourage you to perform due diligence on DOCK5 or any other docking program you choose to use. We certainly do (see McGovern 2003 as above). But I think you also need to have realistic expectations of docking technology. As you point out, getting free energy perturbation calculations to correlate with experiment has been difficult enough. What do you expect with docking calculations that spend a few seconds or even a few minutes per molecule?
Best wishes,
John Irwin http://johnirwin.compbio.ucsf.edu
UCSF
John对计算值与实验值有相关性的评论:如果有人给我看对接能量和实验结合亲和力之间的定量比较,除非它在一个狭窄的SAR系列内(因此不是很有趣),我的直觉是相信这是一个偶然的相关性,并且人们欺骗自己相信这种相关性是显著的。同时也谈到对接虚拟筛选的成功标准:从打分最高的500化合物里买50个化合物进行测试,如果命中3个之前未知活性的化合物,那就是成功了。虽然标准很低,但这是这个领域最顶级的效果了。
侯廷军课题组对多种打分函数的评测
侯廷军课题组的Zhe Wang等人2也考察了10种分子对接软件,结果发现打分值与实验测得的结合亲合力之间的相关性还相当弱(Pearson相关性系数不到0.5,还可能反相关),这说明打分函数不太可靠、通用性也不足。详见博文十种分子对接软件的性能评估3。
D3R第2届竞赛的盲测结果
D3R第2届竞赛结果表明4,所有参赛者提交的预测的结合亲和力与实验结合亲和力的Kendall’s Tau相关性系数不超过0.45(第一阶段竞赛) 与0.46(第二阶段竞赛),这说明预测结合亲和力或对化合物活性排序有很大的提升空间。而代表空假设(没用预测方法的)的油水分配系数cLogP对结合亲和力预测性能表现与参赛者相当,其Kendall’s Tau相关性系数为0.45。
总结
综上所述,分子对接打分值与实验结合亲和力的相关性都不大,分子对接打分值不能用来精确地预测化合物的结合亲和力或精确地排序,但是可以用预测结合模式与富集活性化合物。
参考文献
- Enyedy, I. J., & Egan, W. J. (2008). Can we use docking and scoring for hit-to-lead optimization? Journal of Computer-Aided Molecular Design, 22(3–4), 161–168. https://doi.org/10.1007/s10822-007-9165-4
- Wang, Z.; Sun, H.; Yao, X.; Li, D.; Xu, L.; Li, Y.; Tian, S.; Hou, T. Comprehensive Evaluation of Ten Docking Programs on a Diverse Set of Protein–Ligand Complexes: The Prediction Accuracy of Sampling Power and Scoring Power. Phys. Chem. Chem. Phys. 2016, 18 (18), 12964–12975. https://doi.org/10.1039/C6CP01555G.
- 肖高铿.十种分子对接软件的性能评估. http://blog.molcalx.com.cn/2017/02/08/evaluation-ten-docking-program.html
- Gaieb, Z.; Liu, S.; Gathiaka, S.; Chiu, M.; Yang, H.; Shao, C.; Feher, V. A.; Walters, W. P.; Kuhn, B.; Rudolph, M. G.; et al. D3R Grand Challenge 2: Blind Prediction of Protein–Ligand Poses, Affinity Rankings, and Relative Binding Free Energies. J. Comput. Aided. Mol. Des. 2018, 32 (1), 1–20. https://doi.org/10.1007/s10822-017-0088-4.
相关主题
阅读原文,请点击标题:
- 分子对接假阳性、假阴性产生的原因
- 十种分子对接软件的性能评估
- FLARE案例 | WaterSwap计算BRD4抑制剂的结合自由能
- 认清虚拟筛选中的陷阱
本文通过比较高通量筛选(HTS)与虚拟筛选(VS),讨论了分子对接虚拟筛选产生假阳性与假阴性的原因, 发现不合适的分子内能是假阳性的一个主要原因;通过比较HTS与VS的命中化合物,发现两者可以分别发现不同的化合物,因此两者是互补的;通过对虚拟筛选假阴性化合物的复合物结构分析发现,发现不适合的蛋白结合位点是假阴性的一个重要原因;本文还比较了分子对接与2D相似性的虚拟筛选之间的关系,发现2D相似性方法不能发现竞争性抑制剂与基于结构的方法可以, 由此说明虚拟筛选不同于二维结构相似的方法,可以找到结构新颖的,可逆的竞争性抑制剂。
本文重点讨论了分子对接预测预测结合模式的精度与成功率,还讨论了打分值与结合亲和力的相关性。
本文以几个结构多样的BRD4抑制剂为例,展示了FLARE的WaterSwap模块预测结合自由能的性能。结果表明,WaterSwap可以精确的预测配体的结合自由能。可以用该方法弥补分子对接对化合物排序精度不足的问题。
分子对接精确排序能力不足,但是足够进行虚拟筛选。虚拟筛选的目的是利用蛋白质靶标或已知生物活性配体的相关信息通过计算的方法来识别具有活性的化合物。虚拟筛选有众多的参数设置,潜伏着许多误区,可能会导致虚拟筛选效率低下甚至完全无用。本文试图对虚拟筛选方法存在的问题、缺点、失败和技术陷阱进行分类,从而让用户意识到它们,并在虚拟筛选的过程中对其规避。